Recommendation Engine for streaming services: Putting data to good use
admin
Miscellaneous
June 1, 2021
4 min read
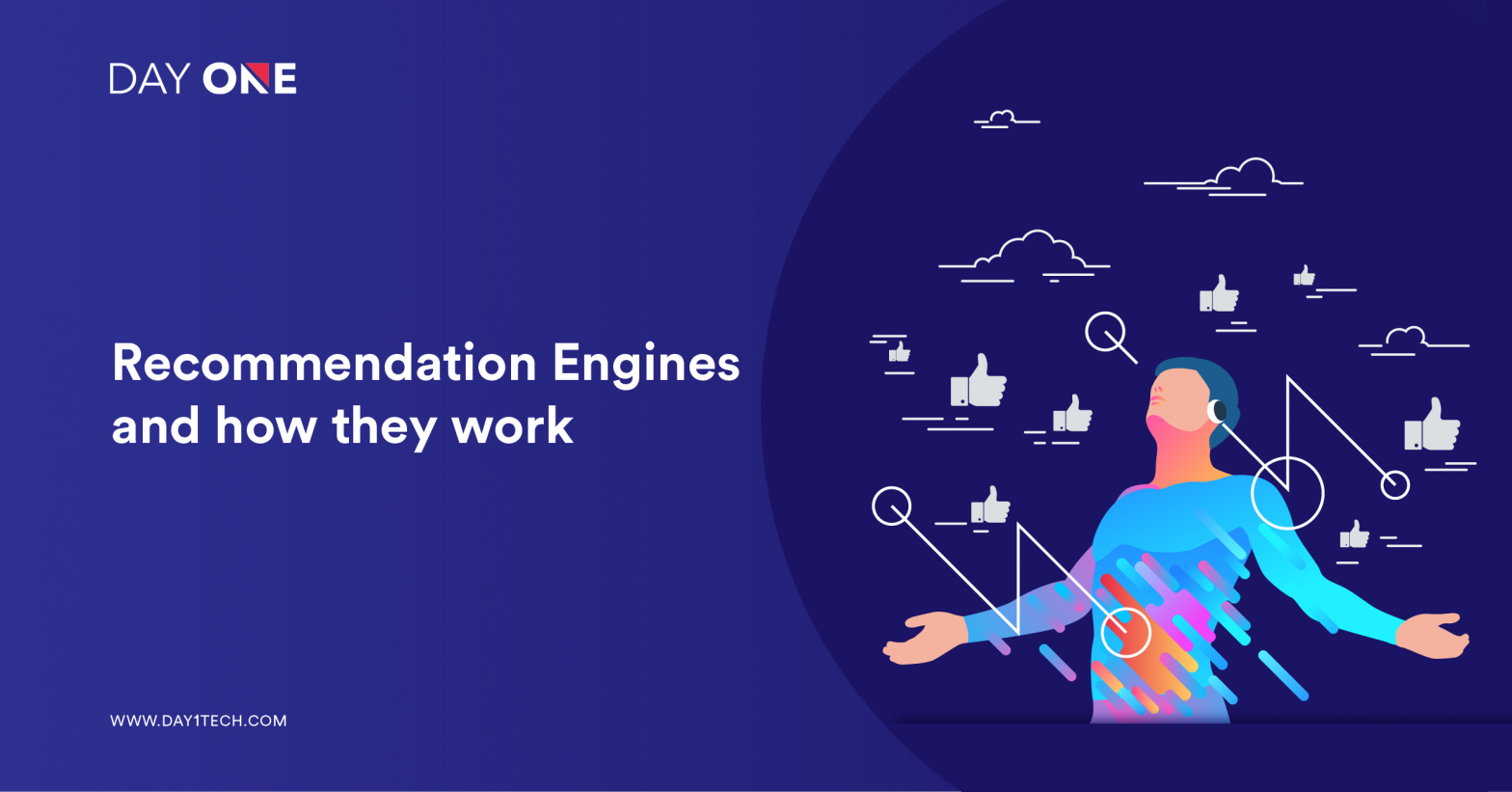
Isn’t Netflix the best partner for you? It is always there for you, shows you things you find interesting, never goes away (unless your internet connection is bad) and remains non-judgemental whether you watch The Avengers or The Witcher. With AI surrounding us in daily life, it is hard not to think of AI especially when it comes to streaming services like YouTube, Spotify and the like.
However, what is lesser known about is how Netflix’s recommendation system works, which makes them have such an insightful journey into the mind of the consumer. Recommendation engines are a vital part of streaming services and the secret sauce to the success of streaming services.
What is a recommendation engine? How do recommendation engines work?
Artificial intelligence has changed the ways of doing business and recommendation engines are one of the most significant applications of AI. Also known as a recommender system, they are algorithms aiming to provide the most relevant and accurate items to the user by filtering products according to the requirements of the consumer as per the information input detected by the algorithm.
If you are wondering “How does the recommendation engine of Netflix work?”, a simple answer would be, by presenting recommendations according to insights based on the actions of the consumer. However, it is also easier said than done. Let us understand how recommendation engines work for streaming services.
A typical recommendation engine consists of four phases namely, collection, storing, analyzing and filtering of data.
- Collection of Data: It goes without saying that any recommendation would require large amounts of data for it to function effectively. So gathering data would be the first step for it to develop.
Data gathered can be either explicit/implicit in nature. Explicit data is the data gathered from the internet from a user’s actions on the internet like ratings, reviews of T.V. shows/movies/songs, etc. Implicit data is data derived from customer actions such as order history, return history, page views, click-through, and other actions. Data can be directly gathered from the user’s actions without requiring any actions from the side of the user. However, huge amounts of data also make it harder to analyze and attain insights.
Since each user has his/her preferences regarding products it will produce unique datasets. As more data input takes place, the recommendation gets smarter buy itself making better suggestions such as “Recommended for You”, the section of Youtube.
- Storage of Data: As we already know recommendation engines require huge amounts of data. The type of data you require helps in selecting the type of storage you require, a no SQL database/ a standard SQL database/ object storage. Each of these can be feasible depending on the user input, ease of implementation, the amount of data storage, integration with the environment, and portability.
- Analyzing the Data: To understand similar skill sets data is filtered by different analysis methods. To provide recommendations to the user, data is analyzed in either of the three forms:
- Data Filtering: To provide the most relevant recommendations, it is necessary to sort the data by filters. Algorithms best suited for the recommendation can be of three types:
Cluster: Recommendations of products that go well together
Content-based: Recommendations of products that have similar properties to what the user likes, e.g., recommending a new trance song playlist to a trance music listener.
Collaborative: Recommendations as per the choices of other similar users.
For the creation of a recommendation engine, massive amounts of data are required. The data is captured from the viewing habits of the consumer. Typical data collected consists of:
- Date and time of viewing the content
- Location and zip code
- Ratings
- Reviews
- Browsing behavior
- When a movie is paused, rewinded or forwarded
Netflix recently tweeted that it’s recommendation algorithm code has driven 75% of it’s viewer activity. Streaming services are developing their algorithms to make their services more personalized. Recommendation engines are helping marketers increase the likelihood of arriving at recommendations tailored to a user’s activity or behavior using in-depth knowledge based on big data analysis.
Types of Recommendations
Usually, recommendations involve three types of filtering:
- Collaborative Filtering: Based on the input of the activities of a user, a user is classified under a certain category. Activities of other people of the same category/similar category are identified and then shows/songs/movies are suggested as per activities of similar users.
User-User Collaborative Filtering: In this type of filtering, preferences of similar audiences are recommended to each other. This kind of recommendation requires computing every customer pair information, which is a time-consuming affair. For example, if Jonathan and Samantha like pop music, then the algorithm would identify the artists Jonathan listens to and recommend them to Samantha and vice-versa.
Item-Item Collaborative Filtering: Similar to user-to-user collaborative filtering, this type of filtering searches for lookalike items. The recommender engine searches lookalike items from the items and recommends them to the consumer.
- Content-Based Filtering: Content recommendation engines work according to the description of items and the preferred choices of the user. The items would carry similar keywords to the other items the consumer has previously consumed. Content-based filtering works on the logic, so Anna likes comedy shows, then the shows in the item matrix which have a keyword description matching comedy will be recommended. Issues remain regarding whether the system is able to learn the preferences of the consumer from their actions regarding one content source and replicate them across different content types. To understand this in an easier manner, if the user expresses interest in sports news, videos related to sports can be suggested, making it much more useful than just presenting sports news.
- Hybrid Recommendation Systems: Combining collaborative and content-based recommendation has been found to be more effective. Several studies have already taken place that proves hybrid approaches would be more effective than a pure collaborative or a content-based approach.
The application of the recommendation system has and is bringing more than billions in revenue. With the application of different types of filtering (content, collaborative and hybrid) the pace and ways of doing business are changing. Today, whether it be Youtube, Spotify or Netflix, all are using these types of filtering so audiences are getting better recommendations and customers are getting a better value for their buck. With the advancements in machine learning, we can safely say, it will make customer retention and loyalty better than ever.
Explore More Blogs
Testimonials What customers have to talk about us
Finch (previously Trio) – Growth with Investing, with benefits of Checking
Reading Time: < 1 minThe Finch (previously Trio), one of our clients today has reached this level with our expertise and with a great team of developers in Day One, who have made every stone unturned in making this project a big success.
Neel Ganu Founder
USA
Vere360 – VR based Immersive Learning
Reading Time: < 1 minDay One helped Vere360 “fill skill gaps” and build a platform that would cater to their niche and diverse audience while seamlessly integrate the best of #AI and #VR technology.
Ms. Adila Sayyed Co-Founder
Singapore
1TAM – Video Blogging Reimagined
Reading Time: < 1 min‘1TAM’ was only for iOS with gesture-based controls, advanced video compression techniques, and a simple architecture that allowed actions to be completed in 2-3 taps. The real challenge for ‘1TAM’ was to keep it distinct which bought brilliant results with all the strategies and approaches implied for best video compression techniques.
Anwar Nusseibeh Founder
UAE
Fit For Work – The Science of Workplace Ergonomics
Reading Time: < 1 minDay One Technologies came with the expertise that was required and helped in building a platform that is edgy, functional, and smart, delivering engagement and conversions at every step.
Ms. Georgina Hannigan Founder
Singapore
SOS Method Meditation for ‘Busy Minds’
Reading Time: < 1 minDay One Technologies helped in building an innovative mobile app (for #iOS and #Android) that’s easy-to-use, engaging, and data-driven to help users reap the most at every point.